We
have built a simulated studio of size (9.2mx8.8m) with office
background. It consists of a living room and a toilet. The living room
is equipped with a bed, a cupboard, a chair and surrounding office
objects. The room could be illuminated by neon lamps on the ceiling or
by sunlight. Based on room layout, we installed four Kinect
sensors at places to observe entire space (see Fig.1). Each Kinect is
plugged to a nearby PC. We note that depth and skeleton information are
not alway available at all positions inside the room.
In
this environment, we collect two datasets. The Dataset-I will capture
activities (including fall and normal activities) of human as in
daily life. So the participants will follow to a pre-defined scenario.
By this way, the actions, mostly falls, will happen at the center field
of view of one Kinect sensor. To evaluate the role of using multiple
Kinect sensors and the role of combining RGB and Skeleton, we collect
the Dataset-II which contain fall and non-fall actitivies happen
at cross-view of two or more Kinect sensors.
Fig1: Environment and material setup
Fig 2: Overlapped views of four Kinect sensors: RGB at the right and
Skeleton at the left. Each color shows the region observed one or more
Kinects. Dataset I
ScenarioWe
capture RGB, Depth and Skeleton data from each Kinect sensor.
These data are calibrated (because depth and RGB sensors of the Kinect
are not the same) then synchronized temporally using Network Time
Protocal (NTP). We define a scenario including daily life and abnormal
activity as follows (see Fig3, Fig4, Fig5, Fig6, Fig7 for illustration).
Illustration of Multiview RGB and Depth (Unsynchronous data)
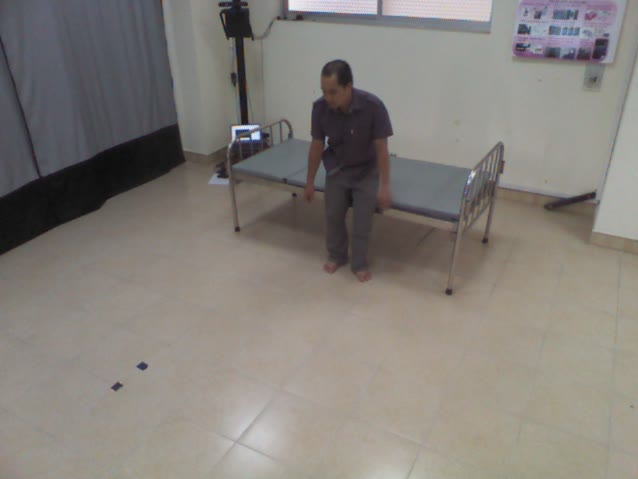 | 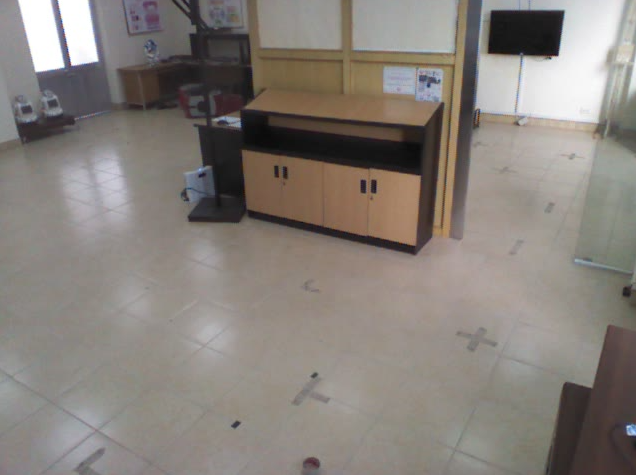 | 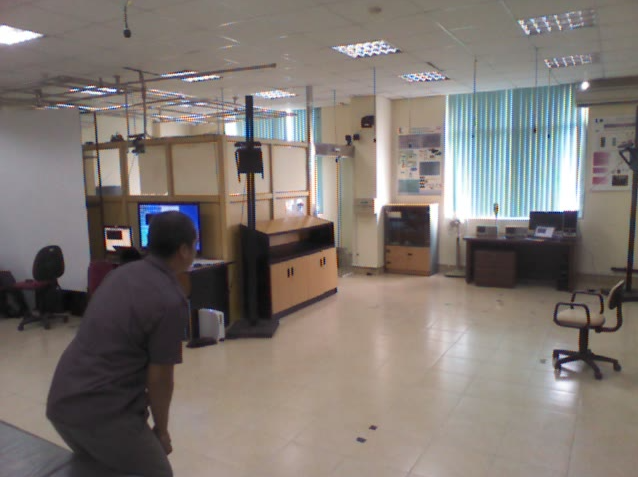
| 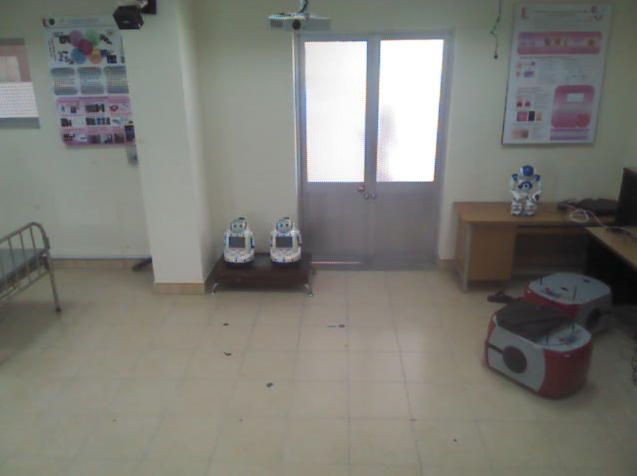 |
Kinect 1 - RGB | Kinect 2 - RGB | Kinect 3 - RGB | Kinect 4 - RGB |
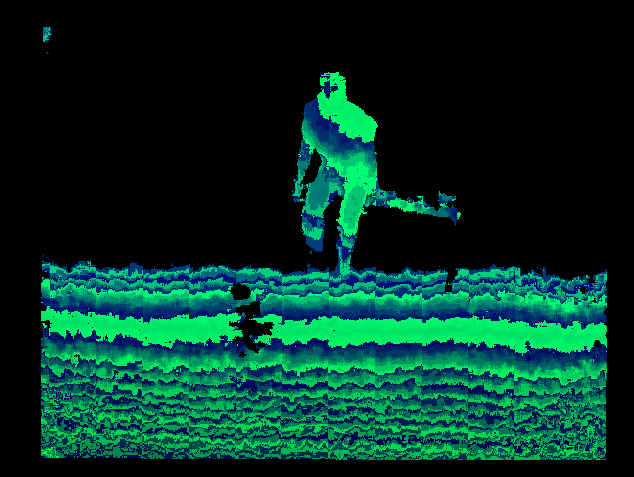 | 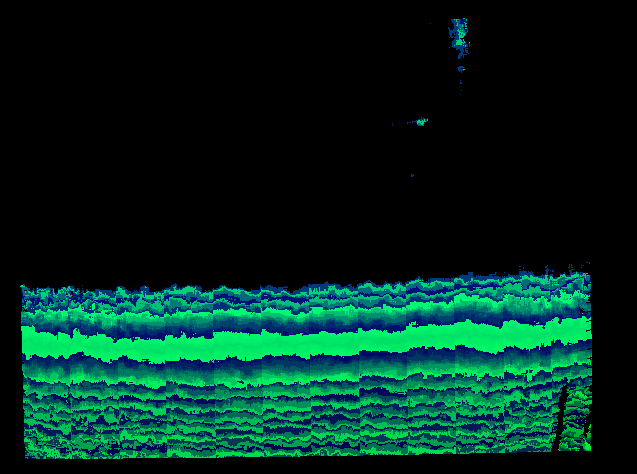 | 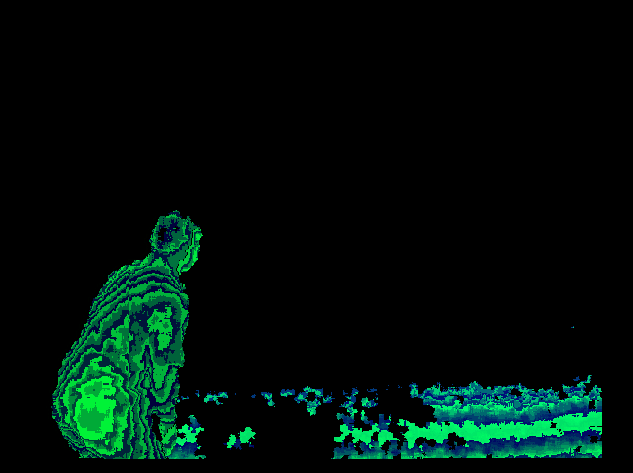
| 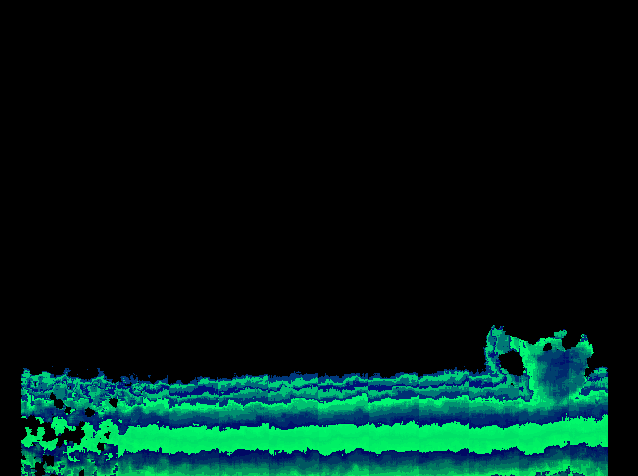 |
Kinect 1 - Depth | Kinect 2 - Depth | Kinect 3 - Depth | Kinect 4 - Depth |
Data collection and storageWe
invite 20 subjects aging from 25 to 35 years old to make 40 falls (20
falls during walking (A12) and 20 falls from bed (A10)) and 200 daily
activities. Daily activities include walking from door to bed (A1);
walking from bed to toilet (A2); walking from toilet to bed (A3);
sitting on the bed (A4); sitting on the chair (A5); lying down the bed
(A6); sitting up from the bed (A7); standing up from the bed (A8);
standing up from the chair (A9); bending and grasping an object (A11).
Totally, the datasets has 80 falls (40 falls from bed and 40 fall
during walking), and other fall-likes or normal activities. Following
is a video of one person playing the whole scenario. RGB, D and
Skeleton captured from 4 Kinect sensors and synchronized data.
All
Kinects are set to 20fps with resolution of 640x480. They capture the
scene at the same time. All data (depth, RGB, skeleton) are then
processed to be synchronous using Network Time Protocol. The dataset
contains 240 videos (20 people x 4 Kinects x 2 data channels (depth,
RGB)), time stamp and skeleton data are stored under text files.
However, only Kinect 1 and Kinect 2 observe fall activities. The size
of the dataset is about 451 Gbytes.
The
data is store in 20 folders corresponding to 20 participants. Each
folder has 4 subdirectories containing data captured from four Kinecs:
Kinect1, Kinect2, Kinect3, Kinect4. Each subdirectory has 2
subsubdirectories containing data from two trials of each person. This
subsubdirectory contains:
- one acceloremeter textfile. This file contains acceloremeter for every frame. (see
example accelerometer file)
- two color and depth videos. These files contain RGB and depth data. (see
example RGB file,
example depth file)
- one skeleton textfile. This file contains coordinates of 20 skeleton joints for every frame. (see
example file)
- one textfile for time synchronization. (see
example file)
Dataset IIThe
main goal of building the Dataset-II is to evaluate the roles of
combining RGB and Skeleton and the role of using multiple Kinects for
fall detection in a large scale space. To this end, we ask subjects to
perform activities (including falls and normal ones) at some specific
positions. To show the role of RGB at unmeasurable range of the Kinect,
falls are performed at positions where the skeleton is not observable
(Fig.2a). To show the advantages of combining multiple Kinects, we ask
participants to perform falls at frontier positions observable by more
than one Kinect (Fig.2b). These positions are very sensitive because
one Kinect could observe only one part of the human body and the whole
action of the human is not observed.
Data collections and storagesWe
invited 4 subjects aging from 30 to 40 years old to make 95
free falls and 206 daily activities (walking, bending, grasping,
jumping, sitting on the chair, sitting on the bed, standing up) at
different positions in these regions. Among the 95 falls during
walking, there are 32 falls at unmeasurable range of all Kinects , so
skeletons are not available; 63 falls at frontier regions observed by
more than two Kinects. The order of performing these activities could
be different from one subject to others and from one trial to another
one.
All
Kinects are set to 20fps with resolution of 640x480. They capture the
scene at the same time. All data (depth, RGB, skeleton) are then
processed to be synchronous using Network Time Protocol. The storage of this dataset is similar to Dataset-I.
DownloadOur datasets (Dataset-I, Dataset-II) are available and free for research purpose. If you want to use our dataset, please contact thanh-hai.tran@mica.edu.vnExample of Fall detection on the dataset (see
falldetectionresults)