Welcome to APSIPA Workshop 2023!
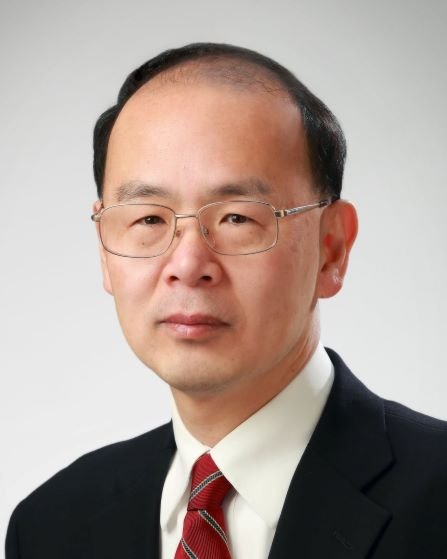
1. Prof. Tatsuya Kawahara
School of Informatics, Kyoto University
Tatsuya Kawahara received B.E. in 1987, M.E. in 1989, and Ph.D. in 1995, all in information science, from Kyoto University, Kyoto, Japan. From 1995 to 1996, he was a Visiting Researcher at Bell Laboratories, Murray Hill, NJ, USA. Currently, he is a Professor and the Dean of the School of Informatics, Kyoto University.
He has published more than 450 academic papers on speech recognition, spoken language processing, and spoken dialogue systems. He has been conducting several projects including speech recognition software Julius, the automatic transcription system deployed in the Japanese Parliament (Diet), and the autonomous android ERICA. From 2003 to 2006, he was a member of IEEE SPS Speech Technical Committee. He was a General Chair of IEEE ASRU 2007. He also served as a Tutorial Chair of INTERSPEECH 2010, a Local Arrangement Chair of ICASSP 2012, and a General Chair of APSIPA ASC 2020. He was an editorial board member of Elsevier Journal of Computer Speech and Language and IEEE/ACM Transactions on Audio, Speech, and Language Processing. From 2018 to 2021, he was the Editor-in-Chief of APSIPA Transactions on Signal and Information Processing. Dr. Kawahara is the President of APSIPA, a board member of ISCA, and a Fellow of IEEE.
Talk: Making a Robot to Communicate with Social Signals
Abstract: Chatbots or dialogue systems have been improved impressively thanks to large language models, but it is not straightforward to implement them into a communicative robot to realize natural spoken dialogue. Among major problems are smooth turn-taking and real-time response without latency. Another important feature is social signals, which provide feedback in a non-lexical manner. It is desirable for a robot to detect them from human speech and also generate them by themselves. We have explored for a human-like social robot ERICA in this direction. Specifically, we have investigated generation of backchannels in appropriate timing, form and prosody, and demonstrated it significantly improves the dialogue experience. We also explore generation of shared laughter, which shows empathy to the dialogue partner. These studies confirm the social signals are fundamental in human communications.
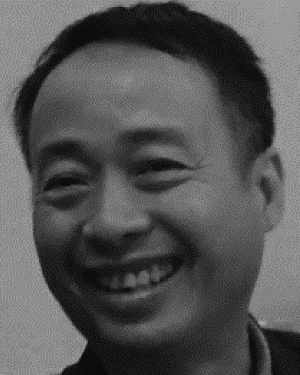
2. Prof. Kosin Chamnongthai
Electronic and Telecommunication Engineering Department, Faculty of Engineering, King Mongkut’s University of Technology Thonburi
KOSIN CHAMNONGTHAI received the B.Eng. degree in applied electronics from the University of Electro-Communications, in 1985, the M.Eng. degree in electrical engineering from the Nippon Institute of Technology, in 1987, and the Ph.D. degree in electrical engineering from Keio University, in 1991.
He is currently a Professor with the Electronic and Telecommunication Engineering Department, Faculty of Engineering, King Mongkut’s University of Technology Thonburi, and a Vice President-Conference of the APSIPA Association, from 2020 to 2023. His research interests include computer vision, image processing, robot vision, signal processing, and pattern recognition. He is a member of IEICE, TESA, ECTI, AIAT, APSIPA, TRS, and EEAAT. He has served as the Chairperson of the IEEE COMSOC Thailand, from 2004 to 2007, and the President of the ECTI Association, from 2018 to 2019. He has served as an Editor for ECTI E-Magazine, from 2011 to 2015, and an Associate Editor for ECTI-EEC Transactions, from 2003 to 2010, and ECTI-CIT Transactions, from 2011 to 2016.
Talk: 3D Point-of-Intention Estimation methods Using Multimodal Fusion of Hand Pointing, Eye Gaze, and Depth Sensing for Collaborate Robots
Abstract: 3D point-of-interest (POI) plays important basic roles for many applications such as intention reading for stroke patients, customer intention estimation, worker intention estimation for collaborate robots, game entertainment, and so on. This talk introduces three methods of 3D POI estimation using eye gazes, multimodal fusion between hand pointing and eye gaze, and multimodal fusion between hand pointing, eye gaze, and depth information for collaborate robots. Based on the nature of human head which is assumed to always move, the 1st method using eye gaze rays finds a 3D POI using a crossing point between a couple of consecutive eye gaze rays detected by an eye tracker, while the 2nd method using multimodal of hand pointing and eye gaze estimates the 3D POI based on a crossing point in the space of interest (SOI) between eye gaze and hand pointing rays in which eye gaze and hand pointing are detected by an eye tracker and a Leap Motion sensor, respectively. On the other hand, the last method assumes a working status between human workers and collaborate robots in a working site where a work piece in the working site is assumed to become an obstacle and interfere the 3D POI estimation. To solve this problem, a depth sensor system is mathematically designed to be added in the 3D POI estimation system to sense all needed views which make the system possible to reconstruct 3D shape of all objects existing in the working site. Then, the 3D POI is determined based on a volume of interest (VOI) which is the 3D crossing space created by eye gaze, hand pointing, and a 3D object reconstructed by depth information. These proposed three methods of 3D POI estimation would be introduced and discussed in their pros and cons in many applications in the talk.
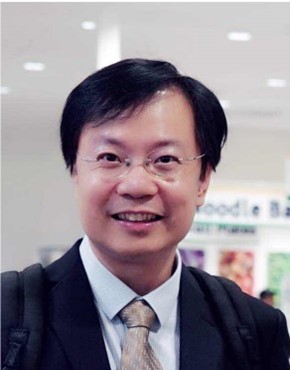
3. Prof. Jing-Ming Guo
Department of Electrical Engineering, National Taiwan University of Science and Technology
Prof. Guo received the Ph.D. degree from the Institute of Communication Engineering, National Taiwan University, Taipei, Taiwan, in 2004. He is currently a full Professor with the Department of Electrical Engineering, and Director of Advanced Intelligent Image and Vision Technology Research Center.
He was Vice Dean of the College of Electrical Engineering and Computer Science, and Director of the Innovative Business Incubation Center, Office of Research and Development. He was Visiting Scholar at the Digital Video and Multimedia Lab, Department of Electrical Engineering, Columbia University, USA from June to August, 2015, and the Signal Processing Lab, Department of Electrical and Computer Engineering, University of California, Santa Barbara, USA from July 2002 to June 2003 and June-November, 2014. His research interests include multimedia signal processing, biometrics, computer vision, and digital halftoning. Dr. Guo is a Senior Member of the IEEE and Fellow of the IET. He has been promoted as a Distinguished Professor in 2012 for his significant research contributions. He has received many awards, including Best Paper Awards (IEEE ICCE-TW 2020, IS3C 2020, NSSSE 2020, ICSSE 2011 and 2020, ICS 2014, and CVGIP 2005, 2006, 2013, 2016, and 2019), Outstanding Contribution Award from Taiwan Consumer Electronics Society in 2021, Outstanding Electrical Engineering Professor Award from Chinese Institute of Electrical Engineering, Taiwan in 2016, Outstanding Research Contribution Award from Institute of System Engineering in 2017, Excellence Research Awards from his university for five times (2008, 2011, 2014, 2017, 2020, and 2022, this award is evaluated and issued every three years), Outstanding Industry-Academia Collaboration Awards from Ministry of Science and Technology, Taiwan, in 2013. Outstanding youth Electrical Engineer Award from Chinese Institute of Electrical Engineering in 2011, Outstanding young Investigator Award from Taiwan Institute of System Engineering in 2011. Dr. Guo is Chapter Chair of IEEE Signal Processing Society, Taipei Section, Board of Governor member of Asia Pacific Signal and Information Processing Association, and President of IET Taipei Local Network. He will be/was General Chair of many international conferences, e.g., APSIPA 2023, IEEE Life Science Workshop 2020, ISPACS 2019, IEEE ICCE-Berlin 2019, IWAIT 2018, and IEEE ICCE-TW 2015. He will be/was Technical Program Chair of many international conferences as well, e.g., IEEE ICIP 2023, IWAIT 2022, IEEE ICCE-TW 2014, IEEE ISCE 2013, and ISPACS 2012. He is/was Associate Editor of the IEEE Transactions on Image Processing, IEEE Transactions on Circuits and Systems for Video Technologies, IEEE Transactions on Multimedia, IEEE Signal Processing Letters, Information Sciences, Signal Processing, and Journal of Information Science and Engineering.
Talk: Trends on the Generative AI
Abstract: This presentation explores the latest trends in Generative AI, focusing on key topics such as the evolution of GPT, expert-level optimization in large language models (LLMs), micro-sizing LLMs, and the deployment of Generative AI in Taiwan's Industrial Technology Research Institute (ITRI) and its potential expansion to Taiwanese enterprises. The talk delves into the advancements in GPT models, highlights the significance of expert-level optimization techniques in enhancing LLM performance, and discusses strategies for scaling down large models. Moreover, it showcases the current efforts by ITRI in implementing Generative AI and outlines the future prospects of adopting this technology within Taiwan's business landscape.
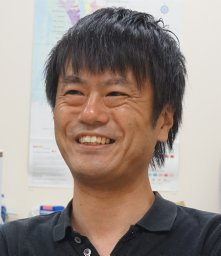
4. Prof. Toshihisa Tanaka
Department of Electrical Engineering, Tokyo University of Agriculture and Technology
Toshihisa Tanaka received the B.E., the M.E., and the Ph.D. degrees from the Tokyo Institute of Technology in 1997, 2000, and 2002, respectively. From 2000 to 2002, he was a JSPS Research Fellow.
From October 2002 to March 2004, he was a Research Scientist at RIKEN Brain Science Institute. In April 2004, he joined the Department of Electrical and Electronic Engineering, at the Tokyo University of Agriculture and Technology, where he is currently a Professor. In 2005, he was a Royal Society visiting fellow at the Communications and Signal Processing Group, Imperial College London, U.K. From June 2011 to October 2011, he was a visiting faculty member in the Department of Electrical Engineering, the University of Hawaii at Manoa. His research interests include a broad area of signal processing and machine learning, including brain and biomedical signal processing, brain-machine interfaces, and adaptive systems. He is a co-editor of Signal Processing Techniques for Knowledge Extraction and Information Fusion (with Mandic, Springer), 2008, and a leading co-editor of Signal Processing and Machine Learning for Brain-Machine Interfaces (with Arvaneh, IET, U.K.), 2018. He served as an associate editor and a guest editor of special issues in journals, including IEEE Access, Neurocomputing, IEICE Transactions on Fundamentals, Computational Intelligence and Neuroscience (Hindawi), IEEE Transactions on Neural Networks and Learning Systems, Applied Sciences (MDPI), and Advances in Data Science and Adaptive Analysis (World Scientific). He served as editor-in-chief of Signals (MDPI). Currently, he serves as an associate editor of Neural Networks (Elsevier). Furthermore, he served as a Member-at-Large, on the Board of Governors (BoG) of the Asia-Pacific Signal and Information Processing Association (APSIPA). He was a Distinguished Lecturer of APSIPA. He serves as a Vice-President of APSIPA. He is a senior member of IEEE, and a member of IEICE, APSIPA, the Society for Neuroscience, and the Japan Epilepsy Society. He is the co-founder and CTO of Sigron, Inc.
Talk: Synthesizing Speech from ECoG with a Combination of Transformer-based Encoder and Neural Vocoder
Abstract: This talk addresses a novel invasive brain–computer interface (BCI) paradigm that has successfully reconstructed spoken sentences from invasive electrocorticogram (ECoG) signals using deep-neural-network-based encoders and a pre-trained neural vocoder. Our group recorded ECoG signals while 13 participants were speaking short sentences. Our BCI could map the ECoG recording to the log-mel spectrograms of the spoken sentences using a bidirectional long short-term memory (BLSTM) or a Transformer. The estimated log-mel spectrograms were used in Parallel Wave-GAN to synthesize speech waveforms. An evaluation of the model performance revealed that the Transformer model significantly outperformed (Wilcoxon signed-rank test, p < 0.001) the BLSTM in terms of mean square error loss and Pearson correlation.